4 Times to Never Use AI in Healthcare
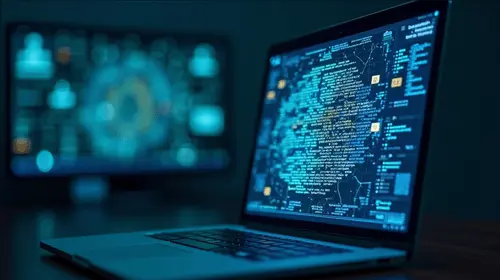
We have a saying in the operating room, “just because you can, doesn't mean you should.” We have microscopic graspers, robots, and laser-guided navigation at our disposal, yet there’s still a doctor behind those tools, making sure everything is running smoothly.
The same principle works for the use of AI in healthcare. There are scenarios where AI is so much better than the current state (I wouldn’t be surprised if we have mandates for AI use in the near future), but there are a few situations that are just not ready for totally autonomous AI applications.
What is AI best suited for? Understanding large volumes of data and making decisions or actions based on clear rules. But context matters. If patient outcomes are at risk, then AI should serve as a copilot, improving the delivery of care but not without human input. In the business world of healthcare, AI can support human decision making to improve efficiencies in the claims processing.
When to Never Use AI in Healthcare
Hearing examples of when to never use AI in healthcare may not be exactly what you were expecting. To clarify, when we say “never” we really mean…not any time soon. With AI improvements in the future, who knows what’s possible. While we’re huge advocates of improving healthcare processes with AI, we’re also huge advocates of transparency. So, let’s cut to the chase with where we stand today.
1. Autonomous Denial Decisions (Care or Claim)
Denials are complex decisions. Whether you’re dealing with a claim or care denial, the decision is too important to hand off to AI—humans still need to be involved. There’s not only a moral responsibility to weigh in on these decisions, but, in instances where AI is used improperly, health plans can face—and are facing—lawsuits, specifically in claims adjudication and prior-authorization determinations that lead to denials. Current lawsuits state that AI auto-denials did not meet the minimum requirements for physician review and that AI was used to deny coverage and care. This is not appropriate.
2. Decision-Making Based on Past Decisions
You can’t trust a system to make decisions based on how it made past decisions because, well, what if those past decisions were incorrect? Not only that, but with policy rules often distributed across systems or imperfectly codified by humans, many AI systems perpetuate an imperfect understanding of policy or attempt to improve decisions based on past successful or unsuccessful recoveries. Neither instance addresses the root problem of a lack of a policy source of truth.
3. Layering AI Onto Legacy Systems
Imagine trying to build a skyscraper on a foundation meant for a three bedroom family home... probably wouldn’t end well. Many health plans data science teams are leveraging AI tools built into enterprise platforms, such as AWS, but failing to understand the complexity of healthcare claims processes. In this case, AI tools for enterprise platforms are the skyscrapers and the claims processes are the unfit foundation. The two don’t gel because there are intricacies of the healthcare claims process that these enterprise tools don’t understand. AI isn’t a one-size-fits-all solution.
4. Models That Rely on Stale Data
AI without learning is like a pencil with two erasers…pointless. AI can get increasingly better at orchestrating tasks, but only if there is a constant feedback loop, helping AI understand what it did incorrectly so it avoids mistakes in the future. Not only is feedback paramount, but ensuring the data that the AI tool is reading is clean and accurate is also critical. Put simply, bad data means bad results. AI is only as good as the data it has access to.
When to Use AI in Healthcare
It’s not all doom and gloom, obviously (otherwise we wouldn’t be in business). There are times when AI can—and should—be introduced into healthcare processes to increase efficiency, improve accuracy, and cut costs.
1. Data Mining to Extract Patterns
One thing that’s certain about the healthcare industry is there is a ton of data. One medical record can contain hundreds, even thousands of pages, collected over years by multiple providers. Rather than having a team of people scour each page for notable patient details that could help inform an insurance claim, AI can be used. Looking for patterns in data is AI’s bread and butter. Then, the data can be summarized and suggested actions can be presented to human reviewers in a way that’s easy to understand and use.
2. Autonomous Payment Decisions Based on Codified Rules
The goal when introducing AI to improve the accuracy of healthcare payment decisions (i.e., payment integrity) is to build and incorporate a set of rules—let's say ones updated by CMS in 2023—to distribute payment in an accurate, but automated way.
Cataloging and ingesting the massive number of policies and rules governing how claims are paid is where AI excels. Like how ChatGPT can generate new content based upon a series of prompts, GenAI has novel applications within healthcare, like transforming policy content from various formats (.DOC, PDF) into machine-readable code to apply these rules across all patient claims.
3. Presenting Summaries & Recommended Actions to Human Experts
Today’s payment integrity processing architecture is a series of systems and vendors, each imperfectly understanding the same policies to attempt to catch errors. AI can do most of the heavy lifting when it comes to reviewing policies, claims, and medical records. It can summarize the information and display an easy-to-read version for human review. Yep, human review. Again, AI is meant to assist and serve as a co-pilot, but not run the show.
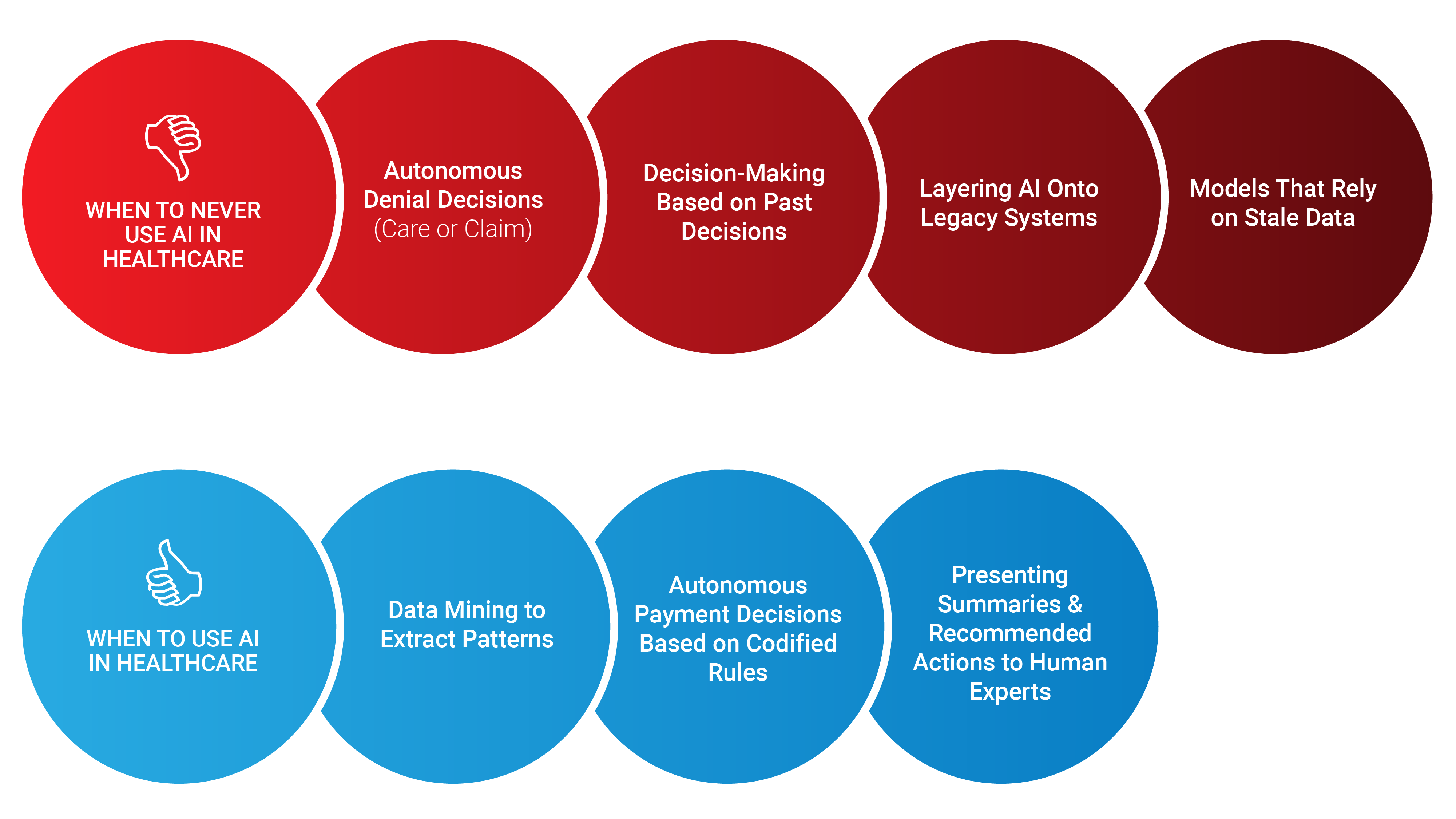
Bringing Safe AI to Healthcare Admin
There’s fear around incorporating AI into healthcare because there’s the assumption of a loss of control—you know, the whole “AI is going to take over” bit. But AI shouldn’t be viewed as a threat. In fact, it should be viewed as a partner. Safe, responsible, and transparent AI-powered software can enable health plans to succeed during this era of healthcare digital transformation by bringing intelligence, automation, and ease-of-use to the healthcare claims lifecycle.
Since Machinify's inception in 2016, we’ve leveraged AI to learn from past administrative decisions to predict the right action to take. Decisions that meet the threshold of safety for AI decision-making are automated while sensitive decisions are handled using integrated review. AI analysis serves as a powerful assistant for validation that can help dramatically increase medical cost savings by 2-3x and boost productivity by over 50%.
To learn more about how Machinify can improve your health plan’s claims processes with AI, schedule a demo today.
This is a selected article for our subscribers
Sign up to our newsletter to read the full version of this article, access our full library and receive relevant news.